Xplain Data’s Causal Discovery algorithms enable companies in all industries to identify crucial hidden causal relationships in their real world data (RWD). By knowing why something is happening, it is possible to target and potentially eliminate causes of errors or achieve a desired effect. Leading enterprises in mechanical engineering, manufacturing, and healthcare use Xplain Data for advanced analyses, production and yield optimization, and care management.
Xplain Data is 100% owned by our founding team.
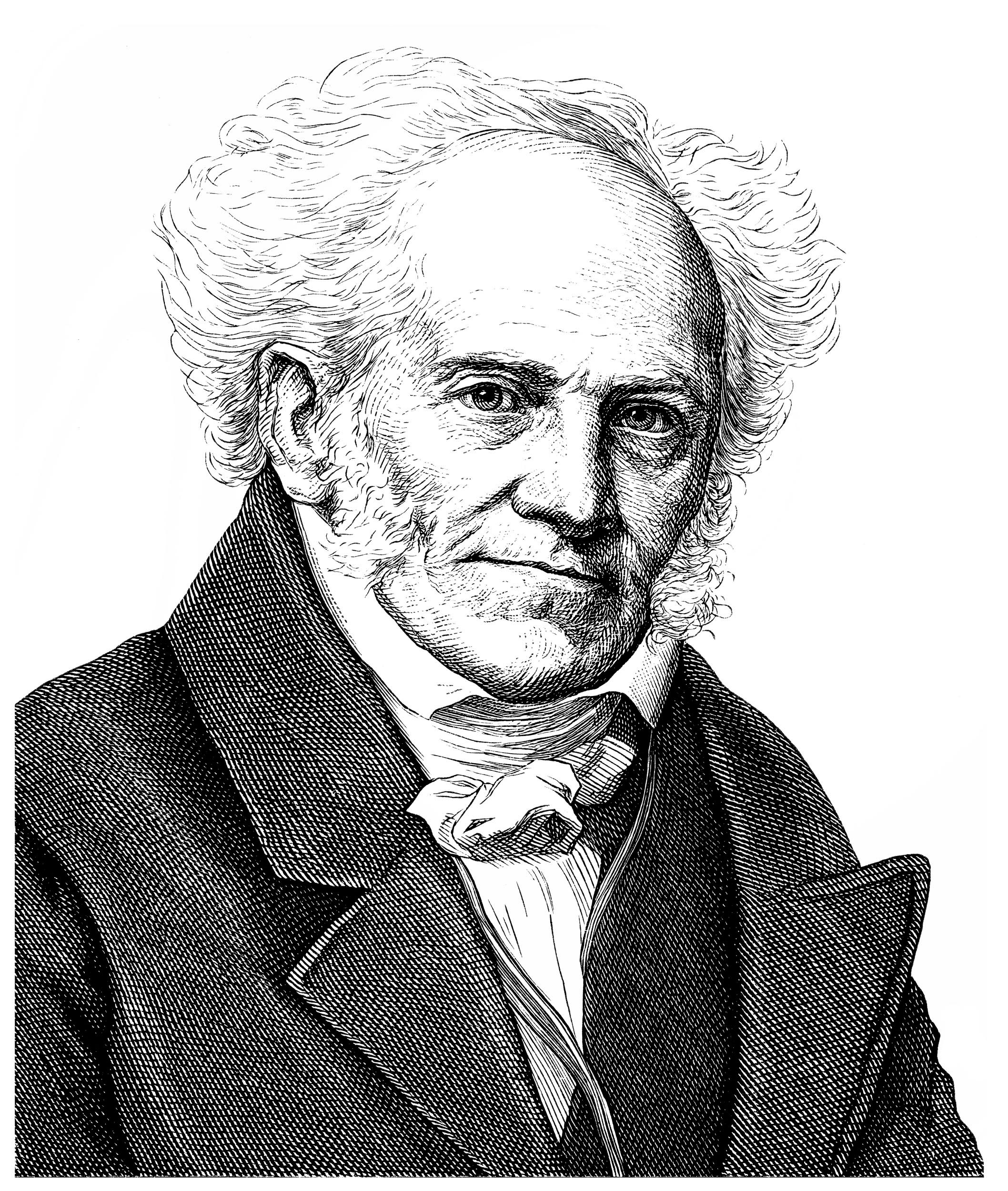
“Each new idea passes through three stages. First, people will ridicule it. Second, it is violently opposed. Finally, it will be considered self-evident.”
– Schopenhauer (1788 – 1860)
Our Approach
In many analytics projects, complex real-world data often clashes with the limited data structures that today’s machine learning algorithms can cope with. Instead of oversimplifying data, we developed a concept to handle complex objects as they are.
Pioneering Big Data and Artificial Intelligence Innovation since 2015
In 2015, we began pioneering innovation in big data and AI, creating the ObjectAnalytics paradigm for analyzing whole objects. This breakthrough advanced a unique path into Causal Discovery.
Innovation through entrepreneurial thinking and collaboration
Innovation thrives on entrepreneurial thinking and collaboration. This we established with our first customers. We welcome visionary partners interested in integrating our breakthrough technologies into their portfolios.
Innovative and empowering
Partnering with us unlocks innovative collaboration models, including our Co-Innovation framework, where risk and reward are shared equally, allowing you to capitalize on breakthrough solutions.
Partner with us!
The buzz about Artificial Intelligence is ubiquitous, but there is no talk about causality. Can a system be intelligent without a notion of cause and effect?
Our Mission
Our mission is to bring viable concepts for causality into the domain of Artificial Intelligence. It requires a holistic view to the object of analysis, which we enable with a novel database technology.
Classic machine learning requires data to fit into constrained analytical schemas, typically a flat table, whereas real-world data is much more complex.
Our Vision
Our vision is to enable Causal AI algorithms that process complex “objects” exactly as they are instead of in an artificially prepared analytics environment.
Want to collaborate with us?
Are you looking for a fresh view on analytics? Are you open to combine the strength of your established company with that of our agile startup?
If so, we are looking forward to hearing from you!