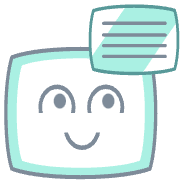
Proactively Safeguard Your Production Line with Continuous Data Analysis
The Causal DiscoveryBot operates seamlessly in the background, scrutinizing data across all production steps. By tirelessly identifying emerging problems and potential causes of failures, it plays a crucial role in securing the integrity of your manufacturing process.
Real-time Alerts for Swift Action: Stay Ahead of Production Challenges
Immediate notifications, delivered through email, Slack, and other channels, keep affected departments and stakeholders in the loop when new causal factors gain significance. This enables a proactive response, ensuring timely countermeasures to maintain the quality and efficiency of your production line.